Transformative AI in Data Handling: Shifting from Traditional Warehousing to Interactive Insights
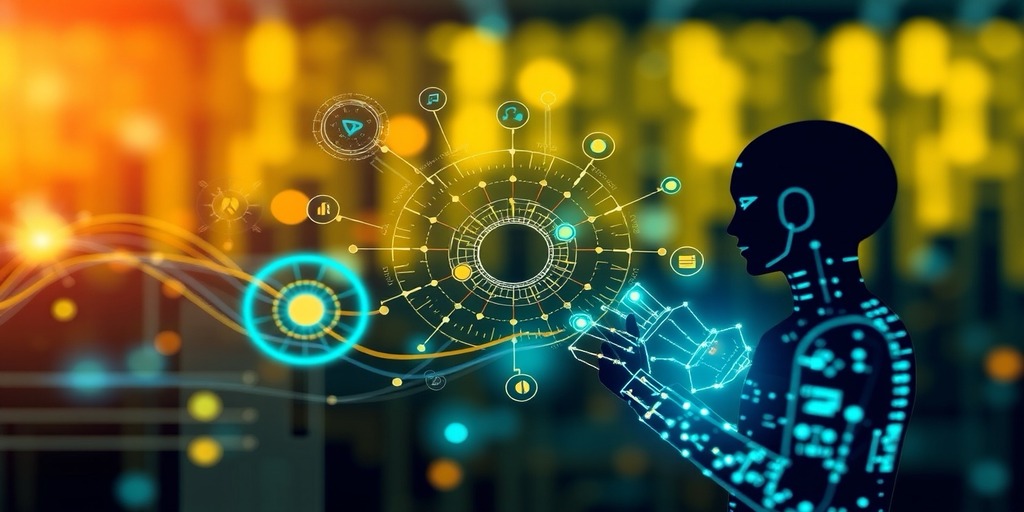
The paradigm of data management is ever changing, for the past two decades we have witnessed a shift from traditional database systems to data warehouses and more recently, to data lakes. Each of these paradigms has brought unique approaches to storing and processing data, reflecting the increasing complexity and volume of information we generate. However, despite these advancements, the fundamental methods of generating insights from data have remained largely unchanged. The data remains to be in a structured format either in its original form or converted to a structured format through ETL/ELT process. Although many may argue Log file and JSON file formats are unstructured, they often follow specific templates that enable some level of structured querying and analysis (semi-structured). With the introduction and advancement of Artificial Intelligence, the landscape is changing once again, significantly altering how businesses perceive and utilize data.
In this blog we will explore the journey from traditional data management to the place where AI-driven insights are taking root , highlighting the benefits, challenges and future prospects.
The Traditional Framework
Data management in the traditional world revolves around the structured data primarily stored in relational databases. This allows efficient querying and analysis through SQL (Structured Query Language). As data volumes grew, organizations sought more robust solutions, which lead to the development of data warehouses. These systems optimized data storage for analytical processing, enabling businesses to generate reports and insights more effectively.
The Emergence of Data Lakes
The change occurred rapidly. As data volumes surged and data warehouses, managed by entities lacking innovation, began to constrain progress, we reached a tipping point. With the advent of distributed file systems and processing, along with the rise of open-source technologies, we experienced significant growth in data lakes. We were now able to store files, this flexibility empowers businesses to store data in its raw form, making it accessible for future analysis without extensive upfront modelling.
Evolution of Data Management Until AI
Data handling has come a long way:
- Early Mainframe Warehouses: Data was stored and processed on mainframes with limited computing capabilities.
- Relational Databases: The introduction of relational databases allowed for more structured data management and faster querying.
- ETL and Data Marts: Data was extracted, transformed, and loaded into specialized data marts for more efficient analysis.
- Structured /Semi-Structured Data: Traditional systems primarily handle data, which is organized in predefined schemas.
- Big Data and Data Lakes: The explosion of unstructured data necessitated scalable solutions like data lakes for storing vast amounts of raw information.
- Cloud Adoption: Cloud-native data warehouses revolutionized the industry with flexible, scalable infrastructure that is easy to deploy and cost-effective.
- Static Reports: The insights generated are often static, requiring manual intervention for deeper analysis.
The Rise of AI-Driven Insights
Today, we stand at the cusp of a new era in data management. AI has revolutionized data handling by introducing advanced techniques such as machine learning (ML), natural language processing (NLP), and predictive analytics. These technologies are changing how businesses perceive and utilize data, shifting the focus from traditional analytical methods to more dynamic and predictive approaches. Machine learning algorithms can now analyse vast datasets in real time, uncovering patterns and insights that were previously hidden. These technologies enable businesses to move beyond static reports to interactive and real-time insights.
Innovative Features Shaping Modern Data Warehousing
- Managing Diverse Data Types
Data is no longer just in tabular or CSV format. Modern data warehouses can handle a wide variety of formats, from unstructured text to columnar, pictorial, and video data. This diversity requires advanced data management capabilities, ensuring that organizations can efficiently store and process data from multiple sources.
Case Insight: Swiggy’s Real-Time Data Needs
Food delivery platforms like Swiggy rely on real-time data to provide a seamless experience for users. Whether it’s updating restaurant availability or tracking deliveries, Swiggy uses a modern data infrastructure that integrates transactional and analytical data in real-time. This helps optimize delivery times and offer personalized user recommendations.
- Real-Time Insights with Hybrid Transactional/Analytical Processing (HTAP)
Hybrid Transactional/Analytical Processing (HTAP) allows organizations to analyse data in real-time without the need for traditional ETL delays. HTAP systems are crucial for businesses that require immediate insights to respond to real-time events, eliminating the need for time-consuming data movement processes.
Case Insight: Leveraging Customer Support Data Marts
In industries like telecommunications, customer support queries are a valuable data source. By creating specialized data marts for different types of queries (e.g., service issues, billing problems), companies can analyse customer interactions in near real-time. This allows support teams to resolve issues faster, improving customer satisfaction.
- Personalization Through Data Warehouses
Personalization is becoming essential in today’s digital age, as customers expect tailored experiences. Modern data warehouses enable businesses to achieve personalization at scale by analysing large volumes of customer data in real-time. From analysing browsing history to identifying patterns in customer behaviour, data warehouses play a central role in delivering customized content and product recommendations.
Case Insight: Spotify’s Real-Time Music Recommendations
Spotify leverages modern data warehousing techniques to deliver personalized music recommendations to its users. By analysing listening habits, song skips, and playlist creation in real-time, Spotify continuously refines its recommendation algorithm. This personalized experience is powered by a sophisticated data infrastructure that supports both real-time analytics and long-term user preferences.
Challenges:
Despite the promising benefits, integrating AI into data management presents several hurdles
- Data Quality: AI algorithms depend on high-quality data; incomplete or inaccurate information can lead to misleading insights.
- Skill Gaps: There is an increasing demand for professionals who possess both data science expertise and domain-specific knowledge to effectively utilize AI tools.
- Ethical Considerations: The implementation of AI raises ethical concerns, particularly regarding data privacy and bias in algorithmic decision-making.
- Integration with Existing Systems: Many organizations face difficulties in seamlessly integrating AI solutions with legacy data management systems, resulting in silos that impede data accessibility.
- Efficiency: Data processing and analysis with reduced time and effort. The efficiency allows businesses to focus on strategic initiatives rather than routine data tasks.
The Future of Data Management
- Data Fabric and Data Mesh : Data Fabric and Data Mesh architectures are becoming popular for managing decentralized data systems. These frameworks allow different teams within an organization to own their data while maintaining a seamless, interconnected system that ensures governance, accessibility, and scalability across the enterprise.
- Augmented Analytics: Combining AI with human intelligence to enhance data analysis and decision-making.
- Edge AI: Processing data at the edge of the network for faster insights and reduced latency.
- Explainable AI: Developing AI systems that provide transparent and understandable insights, fostering trust and adoption.
Looking Forward: AI/ML, Self-Service, and Data Democracy
The future of data warehouses is set to be transformed by AI and machine learning, making data analysis smarter and faster. This means real-time insights and better predictions, boosting efficiency across the board. As companies aim to be more data-driven, self-service analytics and data democracy are key. These tools let everyone, not just the tech experts, dive into data and make informed decisions. Data democracy ensures that insights are accessible to all, fostering collaboration and innovation. However, as we embrace AI, it's crucial to consider ethical implications.
Responsible AI use involves transparency, fairness, and privacy protection, ensuring that data-driven decisions are made ethically. Transitioning from traditional data management to AI-driven insights is a big shift, but the benefits—like improved efficiency, scalability, and personalization—are huge. Embracing this change and fostering a data-driven culture will help businesses unlock their full potential.
We're entering an era where data isn't just stored; it's transformed into actionable insights that drive success, all while maintaining ethical standards.
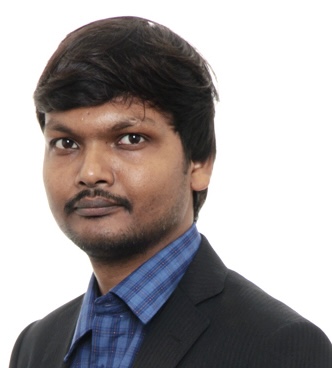
Sivananth Tharmaraj | RIEPL
October 25, 2024
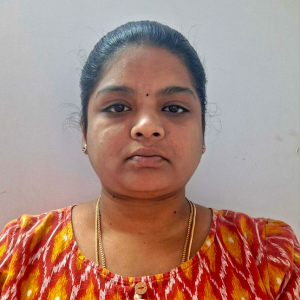
Lakshmipriya Arunachalam | RIEPL
October 25, 2024