Don't Let Your AI Project Fail
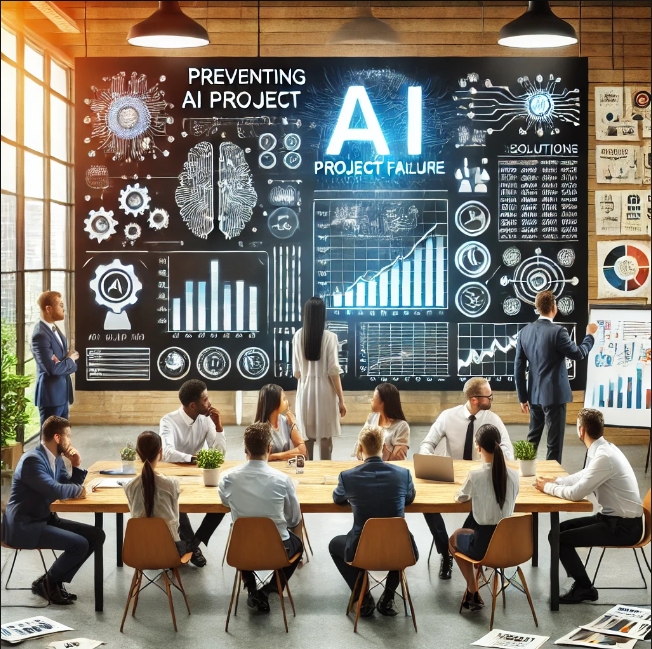
To stay competitive, companies are rapidly moving towards the digital transformations fuelled by Artificial Intelligence (AI). The economic impact caused by the COVID-19 and Geo-political situations has placed an increased pressure on digital transformations to deliver more with less. Companies are re-assessing how to optimise resources and how to best use their budget for maximum value creation, which leaves no room for the failure of any project.
Let’s look some astonishing facts:
- 87% of data science projects never make it into production. (Source: VentureBeat AI report)
- 77% of businesses reports that “business adoption” of big data and AI initiatives continues to represent a big challenge for business. (Source: NewVantage survey )
- 8 out of 10 AI projects had failed while 96% ran into problems with data quality, data labelling, and building model confidence. (Source: Dimensional research report )
- 25% of organizations worldwide that are already using AI solutions report up to 50% failure rate. (Source: IDC )
Factors impacting the success of AI projects:
- Siloed Data: Typically in any company, data is spread across multiple systems and in multiple formats. Many companies lack data infrastructure and do not have enough volume or good quality data. The time and effort needed to improve the data is huge; and AI projects are highly dependent upon the said data, due to which the projects get into a stand still within a short period of time in the need for quality data.
- Skill Sets: The AI powered digital transformation projects need various subject matter experts such as Mathematicians, Statisticians, Data Engineers, Data Scientists. In the absence of right skills, the companies face difficulty in implementing and scaling the projects, which in turn slows down the time to production so the expected business values. Furthermore, many companies cannot afford to recruiting such high skilled manpower and large teams to run these projects.
- Lack of Transparency: Many a times, there are differences in expectations between technical and business teams. For instance, the AI modelling teams may put more focus on model accuracy while business teams give more importance to metrics like, financial benefits, ROI, customer satisfaction, etc. Such mis-alignment between the teams result in failure of AI projects as they try to measure completely different metrics.
- Deployment: For AI projects to create real value for companies, there must be a clear plan on how the project will be used in production; which is often not considered at the time of design and is deferred until the project is completed. This happens because AI teams do not have an architectural view into how their project will be integrated within the production pipeline since these are typically manged by the platform teams. And the platform teams have a very limited insight into the fitment of AI enabled projects into the production environment. Even though the AI project gets completed successfuly but this misalignment may result in a situation of successful projects that don’t deliver expected business value.
- Scalability and Flexibility: Every business undergoes evolution to stay relevant and competitive. This requires a robust architecture that can handle increasing data volumes, and new use cases without the need for significant re-engineering of the system. So, every AI solution must be developed with scalability and flexibility in its core.
- Integration Strategy: To harness the full potential of AI, ease of integration with existing eco-systems is quintessential be it business processes, applications, or data infrastructure. Such integration strategy helps minimize disruption, leverage the strengths of existing infrastructure, and ensure smooth adoption of improvisation and value brought by AI.
Minimising the chances of failure for AI projects: Even though the failure rate of AI projects have been high across the industry, but it doesn’t mean that every project should meet the same fate. There are ways and means to avoid it and enhance the chance of its success.
- Start with a very limited number of business use cases which touch the majority if not all of the existing business process and systems. That is, build MVP first and continuously improve through iterations. This will help to fail faster which is good as it significantly minimises the cost of failure and provide opportunity for necessary corrections.
- Focus on automation. One of the critical challenges for any company is the time needed to complete AI projects which run for many months. AutoML platforms address this by automating manual and iterative steps and enabling project teams to rapidly test new features and validate models. End-to-end automation enables teams to move faster, build and deploy rapidly and make adjustments easily. It also helps companies to explore new features from other data sources. Some of the widely used AutoML tools are: Dataiku, DataRobot, H2O, Enhencer, dotData, Akkio.
- Use white-box models (WBMs). A white box model is a machine learning model used in AI. It provides visibility into the decision-making process, making it easier to identify the key features and rules contributing to predictions. WBMs give not only prediction results but also influencing variables, delivering greater impact to a wider range of stakeholders in AI projects. WBMs are preferred in many enterprise use cases because of their transparent modelling process and easily understandable behaviour.
In conclusion, to succeed companies must leverage AI, robust data infrastructure, automation, to gain more agility and improved accuracy in decision making. The inception of AutoML platforms is helping companies to be more agile and efficient by helping to tap existing resources without having to recruit additional talents.
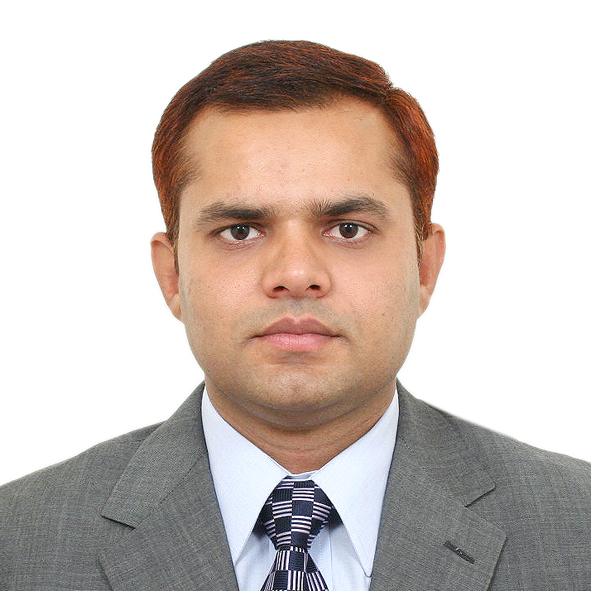
Mantu Tiwari | RIEPL
November 08, 2024