AI-nizing Risk Management in IT Changes
By: Abhijeet Joshi
What is IT Change Management and Why is it Important?
Information technology (IT) forms the foundation of our modern world, driving advancements and supporting daily operations across industries. The critical role of IT is undeniable, and as our reliance on technology continues to grow, the complexity of IT systems also increases. These systems—comprising networks, storage solutions, computer hardware, various software, and countless other digital components—must work together seamlessly to ensure continuous functionality.
To keep these intricate systems operating smoothly year-round, IT teams must regularly implement changes. These changes, known as IT Changes, involve system upgrades, security enhancements, bug fixes, performance optimizations, migrations, and hardware lifecycle management. Ensuring these changes are executed effectively and efficiently is crucial for maintaining optimal performance and controlling costs.
According to ITIL (Information Technology Infrastructure Library) standards, a Change is defined as "the addition, modification, or removal of anything that could have an effect on IT services." IT Change Management is the discipline within ITIL that governs the lifecycle of these Changes, with the primary goal of enabling beneficial Changes while minimizing disruption to IT services.
Why is It Important to Get IT Changes Right?
Any failure or misstep in executing IT Changes can jeopardize critical business operations, leading to revenue loss, damage to brand trust, and, in some cases, legal penalties from regulators. We don’t have to look far for examples of such failures. When CrowdStrike rolled out a faulty software update—an IT Change—it caused widespread issues, disrupting flight operations, banking, healthcare services, and broadcasting. This affected approximately 8.5 million Windows devices globally, with catastrophic consequences for many businesses.
Revolutionizing Change Management with AI
IT practitioners understand these risks, and IT Change Management processes have become highly sophisticated over time. These processes include detailed risk and impact assessments, Change Advisory Board reviews, continuous communication and monitoring, backup and recovery plans, and rigorous compliance practices. However, a fundamental limitation remains: the reliance on human expertise and the risk of process overload.
While AI and machine learning (ML) have been used to build Change Risk prediction models using historical data, these models typically rely only on structured data, missing out on valuable insights hidden in unstructured data sources such as:
- Change descriptions
- Post-implementation reviews, risk assessment feedback, and other notes from previous changes
- Logs and other machine-generated data from impacted systems
- Usage patterns and specifications of applications affected by the changes
- Vulnerability and threat assessments based on publicly available sources
A comprehensive risk assessment model should integrate all these data sources to provide a holistic evaluation of the risks associated with a given change. Conventional ML models excel with structured data, while Large Language Models (LLMs) are now the standard for handling unstructured data. We propose a hybrid approach that combines these two techniques to build a more comprehensive risk assessment model.
Change AI-nization Approach
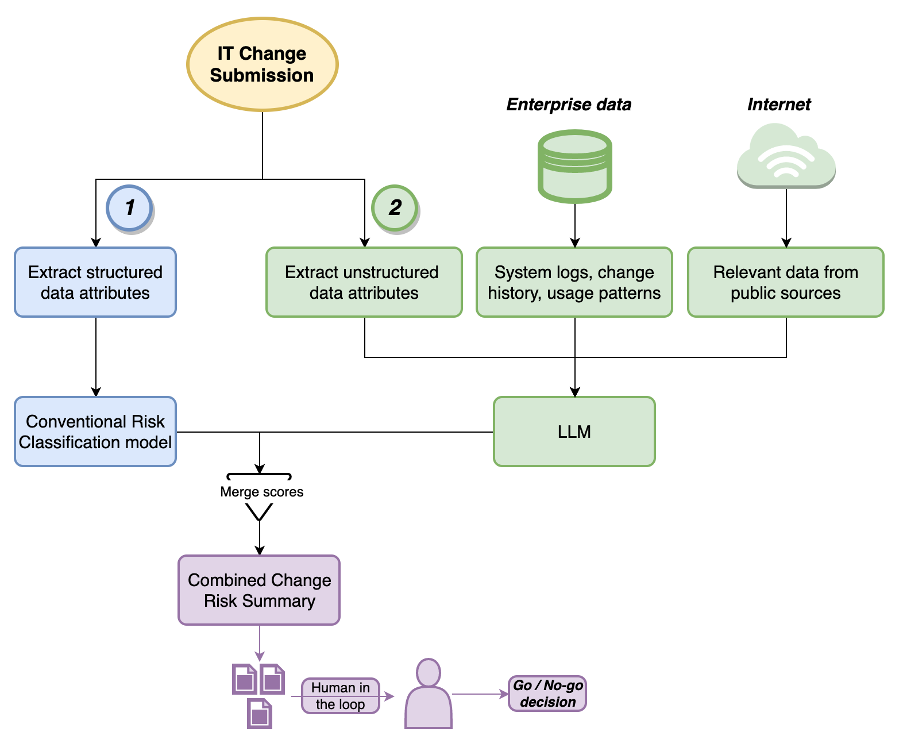
Details of AI-Driven Change Risk Assessment
When a Change is submitted by its creator, the risk assessment process is divided into two parts:
- Structured Data Assessment: All structured data related to the Change is processed through a conventional binary classification model to generate a structured data Risk Score.
- Unstructured Data Assessment: All unstructured data, combined with enterprise data and relevant public sources, is used to generate an unstructured data Risk Score.
These two scores are then combined and presented to the Change Reviewers, who provide a final Go/No-Go assessment for the Change. If a No-Go is determined, the reviewers provide feedback for the Change creators to refine and resubmit. This approach allows reviewers to prioritize their attention on higher-risk changes based on the combined Risk Score.
Benefits of AI-Driven Change Risk Assessment
- Contextual Understanding: The LLM offers a deeper understanding of context, particularly valuable for complex or ambiguous changes.
- Improved Accuracy: Combining a conventional risk classification model with an LLM enhances the overall accuracy of risk prediction by leveraging the strengths of both models.
- Human in the Loop: Change reviewers can focus on decision-making rather than spending time on data collection and risk assessment.
This hybrid approach, combining conventional ML models with LLMs, creates a robust and scalable solution for Change Risk assessment. It provides more accurate risk predictions even in complex scenarios, while improving efficiency for change reviewers. This helps organizations better manage change risks and ensure smoother IT operations.
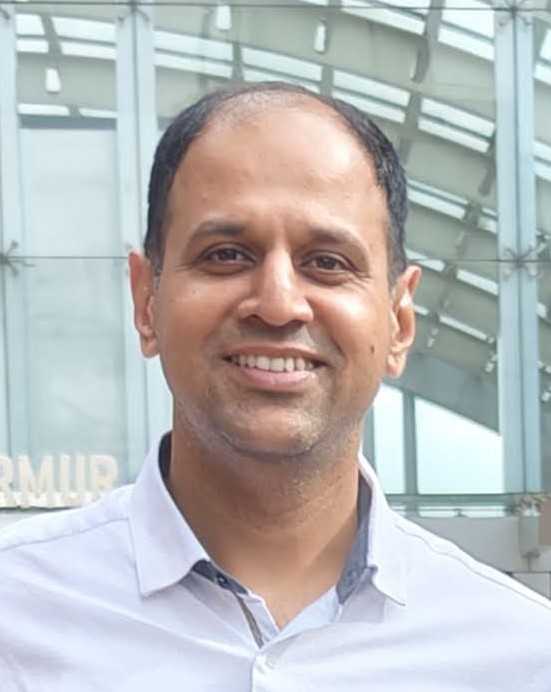
Abhijeet Joshi | RIEPL
August 21, 2024