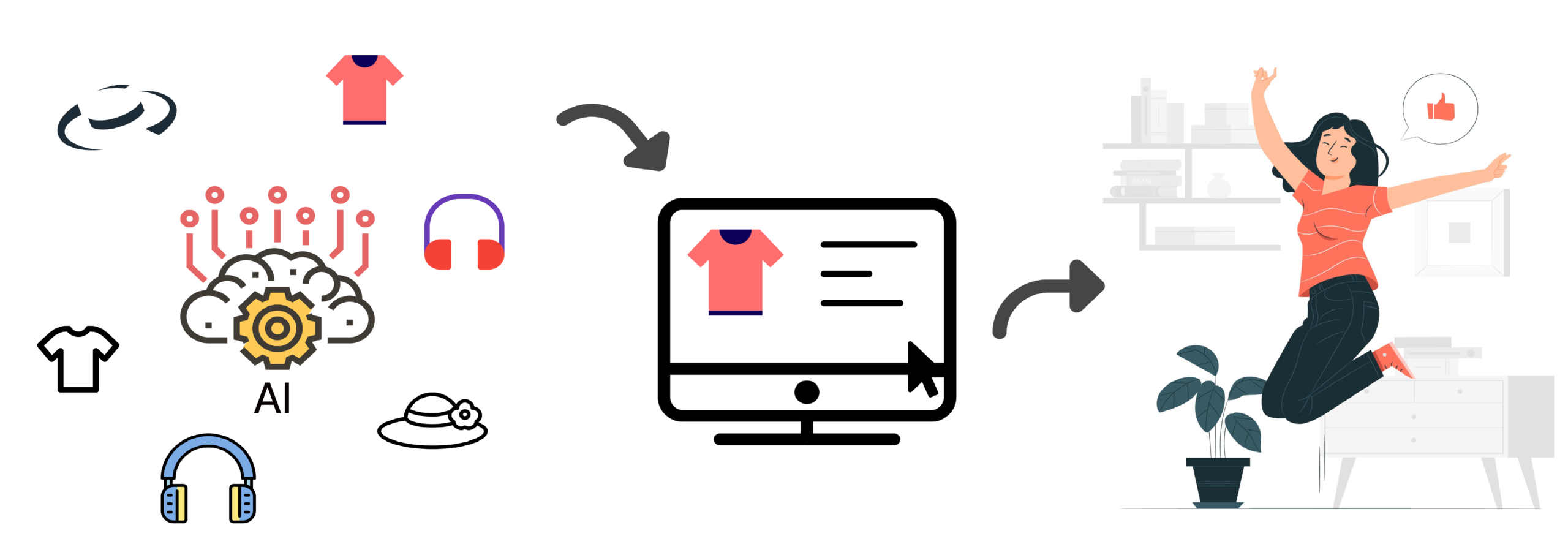
We have all experienced Aha! moments while shopping on eCommerce sites when the product we are searching shows up as a result of our search. Have you ever wondered how?
In this post, I would like to provide a perspective of what typically happens to affect the journey of a product all the way from being an entry in the catalog to the end consumer, i.e. shopper, challenges faced, and how AI techniques help in the journey culminating in shopper delight!
Key Challenges:
- Products involved are in hundreds of millions due to which scalability of solution and cost-effectiveness are paramount.
- Data Quality: Incomplete, missing or incorrect data about products, including a multitude of IDs or the lack thereof.
- Matching products accurately, especially with the flexibility given to merchants/sellers to express product characteristics such as title, product image, etc., leads to non-standardization.
eCommerce catalogs are created by sourcing data from merchants/sellers or manufacturers themselves. To make it easier for sellers to onboard their items, several product characteristics or attributes are not made mandatory. As a result, their product data is often incomplete, missing crucial bits of information that shoppers typically look for. Typically, we find that different sellers tend to provide different product characteristics uploaded onto the catalog. A vast amount of useful data is embedded in the title, product description, and product images which may or may not be characterized as product attributes. The key problem to solve is capturing and characterizing all attributes of a product so that it is indexed appropriately and, in turn, leading to a seamless shopper experience.
When a product item is uploaded into the catalog, we need to check if we already have it. The absence of this would lead to an undesirable shopper experience as the product can show up multiple times in the search result. This requires product recognition and matching to differentiate between a product and its variants, which is fundamental to any eCommerce Catalog.
Ability to discern between products fuels use cases involving an assortment of products that an eCommerce site carries, pricing competitively vis-à-vis competitors, etc. This problem is solved via forming clusters of identical items, typically referred to as `Item Product Clustering’, wherein items that are essentially variants of the product are grouped together. It is imperative that this clustering be accurate, especially in an eCommerce marketplace, as shoppers can choose from different merchants/sellers as they deem appropriate.
Typically, Global Trade Item Number (GTIN) is used for matching and grouping, which is compatible with UPC, JAN, EAN etc. While this will work for the most part, there could be data quality issues with the identifier, which can lead to an incorrect clustering and, in turn, can lead to potentially disastrous consequences based on the product item being sold. To solve this, product matching is done within the catalog based on more tangible product information available, including title, product description, product image, etc., in conjunction with GTIN, if available. Additionally, there are categories such as Fashion wherein items may not have GTIN or other IDs.
Catalog product data enrichment starts via augmenting existing product data with data accumulated from various sources, including manufacturers, third-party providers, etc. Different attribute extraction techniques are employed to extract non-existent or semi-existent attribute information (e.g. brand, colour, size, etc.) from the title and product description using deep learning techniques like Named Entity Recognition (NER) models. To ensure that we are enriching the right product/item, it must be identified accurately before enrichment. Building NER models require the labelling of product information. Given the extensive information involved in dealing with several hundred million products, comprehensive human verification is ruled out except for selective validation. Again this calls for deploying neural network models to estimate similarities.
Another host of models are used to extract and understand images, and a collective decision is made based on product title, description, image, etc. Results from each of these ‘models’ are combined (multimodal) to arrive at match, near match or mismatch decision. Once the product catalog data is enriched, it is sent to the search index to affect the customer search experience (see illustration below).
A lot of sophisticated tooling, data modelling, and representation is put in place to facilitate many of the aspects discussed earlier for developing and deploying deep learning models. Towards illustration, one significant tool is the Annotation Tool which helps in machine labelling and curation by humans and from a powerful data representation Knowledge Graphs used. A Product Knowledge Graph captures knowledge about products and can help provide answers to questions about products. It aids in the discovery of other products, attributes and can power semantic searches, recommendations, etc.
Additionally, having the Product Knowledge Graph aids in deep learning model development and vice-versa. Applied to the Rakuten context, these knowledge graphs can capture customers, services, and products offered by Rakuten, which helps solve use cases at the intersection of product, customer and service knowledge graphs.
Here are a couple of use cases:
- Identifying brand synergies – Which brand(s) of shoes is a user more likely to buy depending on their previous purchases from Uniqlo Jeans and Leica Camera.
- Forecasting consumer’s design and colour preferences (cross-category compatibility forecasting) – for a given set of clothing and electronics a consumer has purchased, what type of design, materials or colours are they likely to choose if they purchased gifts during the holiday season?
We plan to extend the ‘product matching’ concept to do ‘service matching’, ‘merchant matching’ etc., to extend the solutions to businesses beyond eCommerce retail to travel, digital streaming etc. Given that Rakuten Group comprises 100+ companies, it provides a rich ecosystem for solving many such use cases.
Rakuten Product Conference (Theme: Applied AI), scheduled for August 19th and 20th, covers various topics discussed above and much more — covering key areas of customer sciences, analytics and platforms used to build these sophisticated systems and advances in AI. We plan to share our learnings on several relevant aspects as we embark on this challenging yet interesting journey to find that “perfect match” for our consumers while looking to learn from our industry peers on their approaches to solving problems in related industries. On this note, we heartily welcome you to Rakuten Product Conference (Theme: Applied AI).